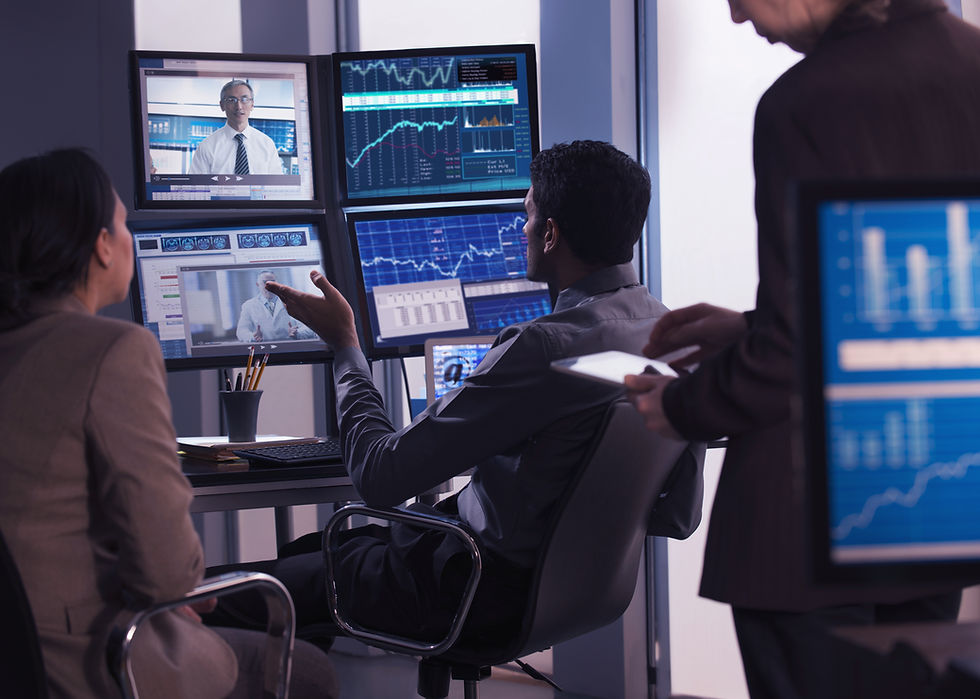
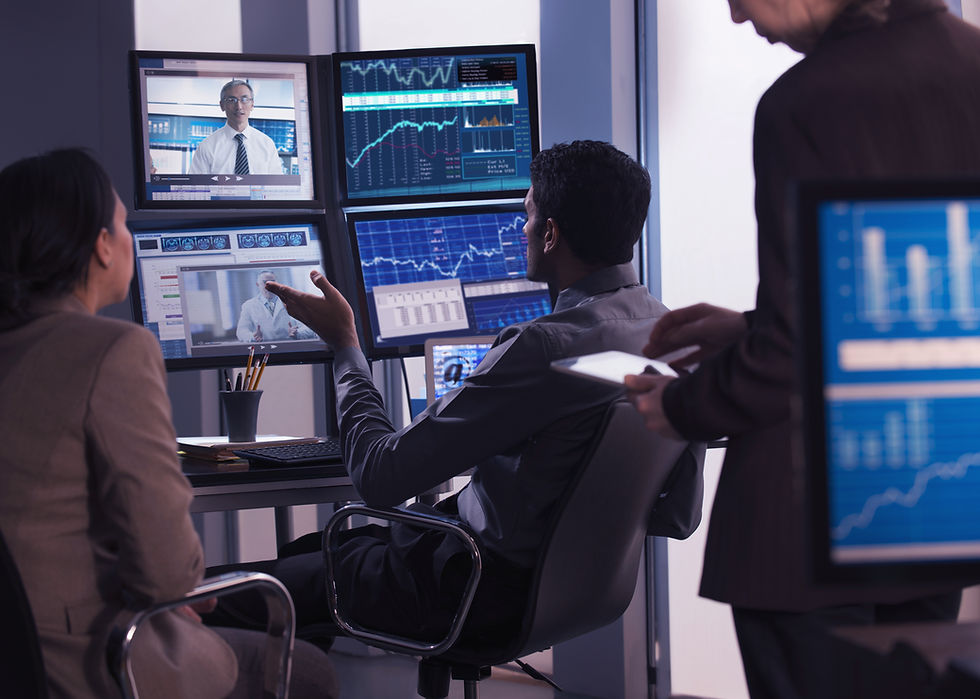
We will cover:
In this workshop, led by world-renowned experts, we will explore practical considerations when building artificial intelligence (AI) and machine learning (ML) solutions for financial institutions. We will provide you with a powerful frameworks and tools to become an expert in your organization.
During the rigorous hands-on exercises, we will internalize best-in-class tools and templates for implementation, maintenance and the buy-in of the your peers and the stakeholders for the artificial intelligence solutions of your choice.
We will also learn how to structurally solve complex legal and compliance challenges arising due to the use of automated decision making systems and artificial intelligence systems.
1. Contrast Top Machine Learning Methods
Sticky, insightful and simple ways of explaining to others the conceptual, mathematical and legal contrasts between the top ML methods: logistic regression, gradient boosting and neural networks and the performance metrics behind them
2. Self-Learn, Self-Execute and Self-Deploy Models: How?
The 'holy-grail' of machine-learning: the self-learning, self-executing and self-deploying models: do they exist at all and, if yes, how to maintain them?
3. Model Building: 'Quick & Dirty' vs. 'Slow & Clean'
When you can build 'quick & dirty' and when you need 'slow & clean' model and why it matters - e.g., why mindlessly focusing on the final ROC value is not good and looking at the ROC curve shape function is often equally important
4. Optimizing your Model Beyond GINI
How to turn-off your autopilot of mindlessly optimizing your models on the standard performance metrics (AUCs, GINIs, loss rate, R2, etc.,), how you can cheat them, why understanding confusion matrix and sensitivity/accuracy/specificity/F1-score is more important and why proper validation design through out-of-time/of-sample tests is a must
5. AI/ML Fit into your IT Architecture
Fitting the IT architecture and platforms (databases, software, tools) into the scope of your AI/ML activities and understanding when Excel is enough (you knew that you can run a neural network in Excel, right?)
6. Optimize for KPIs you Understand
How to ensure that your model is optimized for KPIs you understand, (customer-life-time value, cross/up-sell, churn, expected loss, etc.) and not for some typical data science mumbo jambo mathematical constructs which are often three-degrees removed from your quarterly business targets
7. Proof-of-Value for your A.I. Solution
Getting stakeholders buy-in and board/steerco approvals through sculpting a compelling financial impact analysis for a final go/no-go decision:
how to estimate the marginal added value of your AI/ML solution over other competing solutions through backward- and forward-looking what-if-and the NPV (net present value)- scenarios
modelling across number of scenarios such as with vs. without historic financial data (existing/innovative solution) or record- vs. portfolio- level estimations (slow/fast execution)
8. In-House, Buy or Partner for A.I. ?
Deciding between: in-house developments, hiring new staff, in/outsourcing, buying software, partnering up, creating joint ventures, and acquiring start-ups and hence:
covering possible implementation scenarios on - existing infrastructure, micro-services, internal/external cloud, SaaS, etc.
understanding legal remedies and warranties/guaranties on commercial vs. open-source software
9. End-to-end (E2E) cycles of building, deploying and maintaining robust ML model
Best-in-the class practices of end-to-end (E2E) cycles of building, deploying and maintaining robust ML models across your organizations: why everyone wants always to build the models but none ever wants to implement and maintain them
10. Why most pilots and POCs become final solutions?
Why most pilots and POCs become final solutions and how to stagger and phase them correctly at your own organization or on-site at clients
11. De-biasing algorithms and making them fair again
De-biasing algorithms and making them fair again: managing the model's likely discriminatory misjudgments, about such sensitive user-characteristics as gender, ethnicity, religion or age, which mainly stem from the ill-thought mathematical assumptions and lack of explicit rules for tackling model's hidden correlations
12. How to explain your model's decisions in a compelling way ?
How to explain your model's decisions in a compelling way to the end-users in a compliant manner but without revealing the inner workings of the model and what the current legislation actually mandates so you don't throw the baby out with the bathwater
13. Who is end-responsible for the data processing - you or the party you engage?
Who is end-responsible for the data processing - you or the party you engage? The crucial difference between the data controller, data processor and how to identify them. Is possible to indemnify oneself against GDPR fines by holding accountable the data processor or data controller, can you split the fine or buy an insurance against it?
14. Methods of anonymizing data
Best-in-the-class and industry-standard ways of how to anonymize your data and what is a difference between fully anonymized data and pseudo-anonymized data ?
15. Biometric and sensitive data
Is it worth processing biometric or sensitive personal data ?
Disclaimer
Any views or opinions presented in this workshop are solely those of the author and do not necessarily represent those of the organizations.
Who Will Deliver this Workshop?
Industry Leaders
Peter Lewinski, PhD
Ervaring: Bankieren, Fintech
Peter hielp bij het vinden van een diepgaande SaaS A.I. startup, werkte in startups, banken, fintechs en universiteiten in Londen, Amsterdam, Madrid, Leuven, Zwitserland en Warschau. Hij ontving een driejarige Marie Sklodowska-Curie Fellowship (Europese Commissie) van +250.000 EUR om onderzoek te doen naar consumentengedrag met behulp van A.I. oplossingen.
In het bankdomein heeft hij ervaring opgedaan als adjunct-directeur van de afdeling Credit Risk Modelling bij ING Bank, waar hij een team van 10 data-analisten leidde bij het bouwen van in-house machine learning / A.I. oplossingen van interne en alternatieve big data-bronnen evenals gescout, gekozen en geïmplementeerde fintech start-ups (bijv. twisto.cz, finansowaniefaktur.pl). In fintech-domein heeft hij ervaring opgedaan als programmadirecteur bij Oxford Fintech & SmartLaw Society en als Summer Associate in een op London Mayfair gebaseerd alternatief kredietrisico scorend fintech FriendlyScore (friendlyscore.com).
Vanaf september 2018 trad hij toe tot een toonaangevende boetiek A.I. softwarehuis - smr.nl, waar hij in zijn nieuwe rol als lid van het managementteam verantwoordelijk is voor de strategie en bedrijfsontwikkeling van de eigen deep learning- en machine learning-oplossingen, al geïmplementeerd bij Fortune 500, financiële instellingen, wetshandhavingsinstanties, Ivy League-universiteiten en startups.
Peter is afgestudeerd aan de universiteit van Oxford. Hij promoveerde aan de Universiteit van Amsterdam, was postdoc aan de Université de Neuchâtel en was universitair docent tenure-track aan de ESCP Europe (Warsaw Campus, Kozminski International Business School). Sinds 2011 heeft Peter gesproken op meer dan 20 internationale conferenties, co-auteur van +12 publicaties, zijn wetenschappelijk werk over gedragseconomie is honderden keren geciteerd en ontving talloze beurzen (Europese Commissie, Raad van Europa, Dutch Science Foundation).

Pieter Bision
Ervaring: Bankieren, Fraude, Criminaliteit
Pieter Bison is een ervaren zakenman die altijd op zoek is naar de volgende grote uitdaging. Hij heeft ons in 2003 geholpen bij het oprichten van ons bedrijf. Hij heeft meer dan 20 jaar ervaring in datamining. Hij leverde A.I. / KPI's voor datamining naar klanten in zaken met betrekking tot bankzaken, verzekeringen en fraude / criminaliteit. Hij heeft programmeervaardigheden in meerdere talen, IT-vaardigheden, platforms, cursussen certificaat en krant vermeldingen en prijzen.
Met zijn passie en expertise is het Pieter gelukt om ons bedrijf naar nieuwe hoogtepunten te brengen en groei te realiseren. Bekijk onze contactgegevens voor lezingen of persmogelijkheden met Pieter Bison.
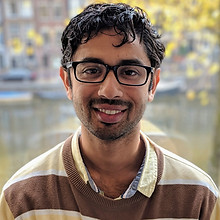
Amogh Gudi
Ervaring: Deep neural networks
Amogh is een onderzoekstechnicus voor machine learning en hij heeft meer dan 5 jaar werkervaring in diepe neurale netwerken. Sinds 2013 werkt Amogh in een Nederlandse A.I. start-up, waar hij heeft gewerkt aan projecten zoals het herkennen van semantische functies met behulp van diep leren of zwak begeleide objectlokalisatie. Daarvoor was hij lid van het Nederlandse Nao-team en behaalde de 3e plaats op Iran Open 2013 - Teheran en top-16 finish in 2013 - Eindhoven. Hij was ook een systeemingenieur voor Tata Consultancy Services.
Amogh is een volleerd wetenschapper. In 2019 promoveert hij op data-rekenefficiëntie in diepe neurale netwerken van de Technische Universiteit Delft. In 2014 behaalde hij zijn Masters in kunstmatige intelligentie aan de Universiteit van Amsterdam en een Bachelor of Engineering aan de Technische Universiteit. Zijn werk is honderd keer geciteerd en hij heeft op tientallen internationale conferenties gepresenteerd.
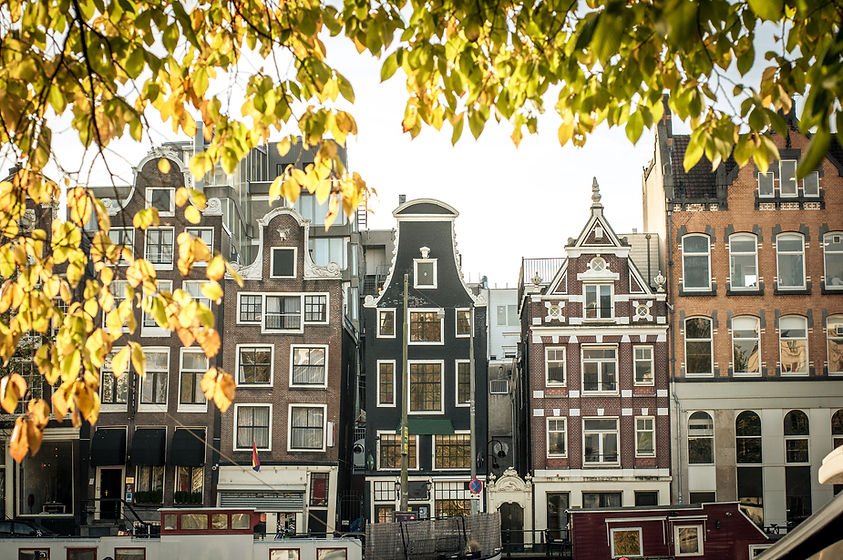
Training
Ga met ons in zee
Bij Sentient zijn we gepassioneerd door leidinggevenden te trainen die hun branche dan blijven verstoren. Sinds 2003 bieden we workshops van hoge kwaliteit aan al onze klanten en naarmate we groeien, blijft onze toewijding aan uw behoeften de belangrijkste drijvende kracht achter onze software startup. Ons team van professionals is hier om u te inspireren met hun unieke ideeën en vaardigheden - neem vandaag nog contact op voor meer informatie.